The Future of Transportation: The Importance of Training Data for Self-Driving Cars
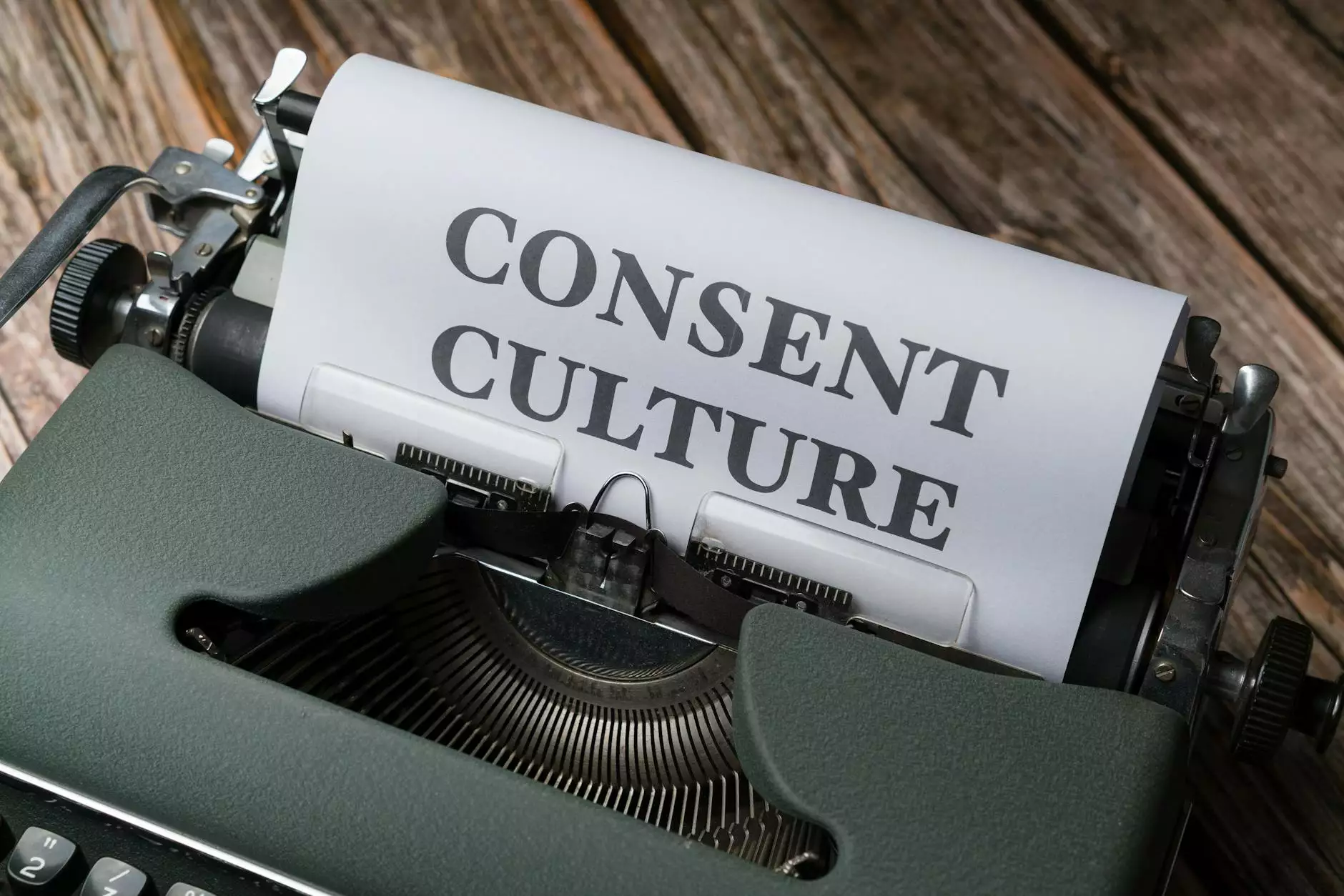
As we stand on the precipice of a new era in transportation, self-driving cars are no longer a distant fantasy but an impending reality. At the heart of this technological evolution lies a crucial element: training data for self-driving cars. This data powers the algorithms that enable autonomous vehicles to navigate, make decisions, and ensure passenger safety. In this comprehensive article, we will explore the significance of training data, its sources, application in real-world scenarios, and its transformative potential for the automotive industry.
Understanding Training Data for Self-Driving Cars
Training data is the bedrock of machine learning, particularly in the sphere of automated driving systems. It consists of vast amounts of information collected from various sources, including cameras, LiDAR, radars, and real-world driving experiences. This data is processed to teach self-driving cars to recognize their surroundings, understand traffic laws, and effectively respond to emergency situations.
The Components of Training Data
The training data encompasses various types of information essential for the functioning of autonomous vehicles:
- Visual Data: Images and videos captured from different angles and lighting conditions.
- Sensor Data: Information from radar and LiDAR sensors for detecting obstacles and mapping the environment.
- Behavioral Data: Insights into human driving behavior, speed patterns, and decision-making nuances.
- Geographical Data: Maps and location specifics to provide context for navigation and positioning.
The Collection of Training Data
Collecting training data involves advanced technologies and methodologies that ensure comprehensive and diverse data. Here are some methods utilized to gather this crucial data:
1. Real-World Driving
Self-driving car manufacturers deploy vehicles equipped with sensors and cameras in urban and rural habitats. These vehicles collect real-time data as they navigate streets under various conditions, ensuring an extensive dataset that reflects real-world scenarios.
2. Simulation Environments
Virtual simulations play a pivotal role in augmenting real-world data. By creating controlled environments, developers can safely test scenarios that are difficult or dangerous to replicate on public roads, such as extreme weather or complex traffic conditions.
3. Public Data Repositories
Many organizations and research institutions publish datasets that automotive engineers can utilize. This open access to data fosters collaboration and accelerates innovation within the self-driving domain.
The Training Process Explained
Once the data is collected, it must be processed and refined to present it in a suitable format for training algorithms. This extensive process initiates several key phases:
Data Labeling
Labeling involves tagging the collected data so that machines can interpret it effectively. For instance, labeling may include identifying vehicles, pedestrians, stop signs, and lane markings in images; this allows the algorithms to understand what these elements represent in driving scenarios.
Model Training
The labeled data is then fed into machine learning models, most commonly neural networks. Through iterative learning, these models adjust their parameters to minimize errors in decision-making, enhancing the overall performance of the self-driving system.
Validation and Testing
After training, the models undergo rigorous testing using separate validation datasets to evaluate their performance. This step is critical to ensure that the self-driving algorithms can generalize well to new and unseen data, which is imperative for safety.
The Impact of Training Data on Safety and Reliability
Perhaps the most significant advantage of high-quality training data is the enhanced safety it offers. By capturing a diverse array of scenarios, autonomous vehicles can learn to handle unexpected situations, significantly decreasing the likelihood of accidents.
Reducing Human Error
Human error is the leading cause of traffic accidents worldwide. By leveraging AI and machine learning, self-driving technology can potentially reduce this risk. As the systems become more adept through extensive training, we can expect a marked decline in mishaps caused by distractions, fatigue, or poor judgment.
Training Data and Urban Mobility
Self-driving cars hold the potential to transform urban mobility dramatically. With efficient algorithms trained on real-world data, autonomous vehicles can navigate crowded city streets, optimizing routes and minimizing congestion. As a result, we can anticipate:
- Reduced Traffic: AI can help manage vehicle flow and minimize bottlenecks.
- Enhanced Accessibility: Self-driving cars can provide mobility solutions for those unable to drive.
- Lower Emissions: Efficient driving patterns lead to reduced fuel consumption and pollutants.
The Future of Training Data in Autonomous Vehicles
The evolution of training data for self-driving cars is an ongoing journey marked by technological advancements and increased data volumes. As the automotive industry continues to invest heavily in autonomous systems, we can look forward to several emerging trends:
1. More Comprehensive Datasets
As autonomous vehicles become more prevalent, the datasets will grow immensely, incorporating data from a wider range of environments, including rural areas, various weather conditions, and diverse driving behaviors.
2. Enhanced Privacy and Ethics
As data collection increases, concerns surrounding privacy and data ethics will become more pronounced. The industry must implement robust measures to ensure that data is collected and used responsibly.
3. Collaboration Across Industries
We will likely see increased collaboration between automotive manufacturers, technology companies, and regulatory bodies to develop standardized approaches to data management and safety protocols.
Conclusion: The Road Ahead
In conclusion, training data for self-driving cars is not just a component of technological advancement; it is the lifeblood that fuels the future of transportation. By investing in comprehensive data collection and analysis, we can unlock a new paradigm in mobility, characterized by enhanced safety, efficiency, and accessibility. As we move forward, it's critical that businesses, such as KeyMakr, stay attuned to these advancements, recognizing the interconnectedness of technology and the services we provide. Emphasizing these trends will ensure a competitive edge in this rapidly evolving landscape, ultimately benefiting consumers, industries, and society as a whole.
As we embrace the future of autonomous driving, the importance of quality training data will only intensify, propelling us toward a safer, smarter, and more sustainable world.
training data for self driving cars